SynthEye
Generating high-quality synthetic images using Latent Diffusion Models
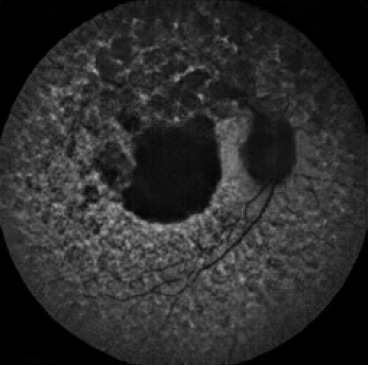
Background:
Diagnosis of rare diseases is challenging in medical imaging due to the natural class imbalance in datasets, which causes models to overpredict the majority class.
Project proposal:
Deep generative models belong to a class of deep learning models that model the distribution that produces the data examples. This is challenging due to the high-dimensional nature of images. Various models have been developed that tackle this problem, including generative adversarial networks, variational autoencoders, autoregressive models, and flow-based models. Recently, diffusion models have taken the storm in the AI community due to their ability to model data distribution and also generate high-quality examples. While these models have been successful in synthesizing natural images, the generation of medical images, particularly pathological ophthalmic images, is relatively underexplored. This study will explore the synthetic generation of images of retinal diseases using diffusion models.
Dataset:
Through our connection to Moorfields Eye Hospital, our lab has access to extremely large ophthalmic imaging datasets for color fundus photography, optical coherence tomography, and infrared imaging. The choice of the dataset is up to the student.
Goals:
- The interested student can implement a basic denoising diffusion model on a publicly available natural images dataset.
- The model can be trained specifically for retinal images.
- This approach can be extended to conditional image generation of specific diseases.
- Latent diffusion models would be the next point of exploration for conditional image generation.
- On the side, the student must also implement approaches for image quality evaluation in order to validate that the synthetic images are of reasonable quality.
Some applications
- Decision-boundary aware data augmentation for imbalanced image classification
- Model a retina picture from first principles or images from literature or UK Biobank
- Myopia oct transformation (morphing of retina).
- Age augmentation, can we age a retina synthetically?
- Ethnicity augmentation, can we transform across ethnicities?
- Generate disease images (e.g introduce edemas)
- Synthetic nevus generator
- Standardisation of Optos (e.g dealing with the artifactual colouring)
- Colour fundus to Optos or vice-versa