EyeCon
Decision support systems for keratoconus
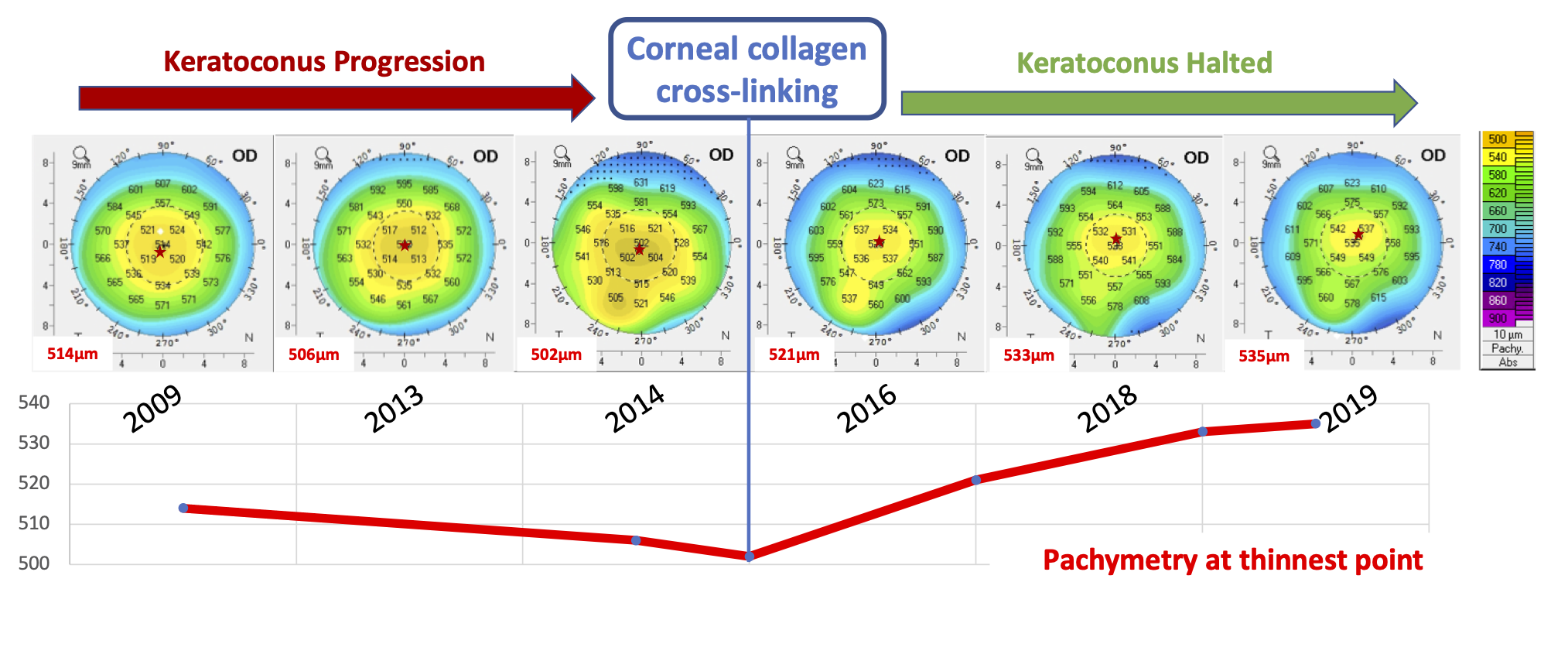
Keratoconus
Keratoconus is a bilateral ectatic disease of the cornea that can cause visual loss through corneal distortion and scarring. It is a leading cause for visual impairment in adolescents and young adults, second only to myopia and simple astigmatism. The prevalence of keratoconus varies from 1 in 375 in North Europe to as high as 1 in 48 in some ethnic groups.
Early Detection
The majority of individuals with keratoconus are identified because of symptoms of visual disturbance or an increase in astigmatism at refraction. Individuals with keratoconus are often detected too late, at a stage when visual deterioration has already occurred. The detection of keratoconus at an early stage has become increasingly relevant since the introduction of corneal collagen cross-linking (CXL) which can arrest progression of keratoconus in 98.3% of eyes. Given the existence of a cost effective and low risk treatment, the ability to identify individuals who are at high risk of developing keratoconus would be a significant advance in clinical care with public health implications. Machine learning has the ability to synthesise the large number of parameters that can be derived from corneal imaging as well as other clinical and biometric measures such as visual acuity and refraction. It may offer superior diagnostic ability which when implemented as an automated screening tool, could result in remarkable advances in case detection, mitigating both expenditure on new imaging hardware and the existing burden on ophthalmic health care professionals. However, diagnosing very early forms of keratoconus (subclinical keratoconus, forme fruste keratoconus or suspect keratoconus) remains a challenging problem due to both ambiguity in its definition and the subtle anatomical changes inherent to this condition.
New Avenues of Research
Despite the growing literature on the diagnosis of subclinical keratoconus, there is a need for further fundamental research, particularly for analysis based on the raw pixel values. Our team hope to develop a multimodal solution by combining raw images with other parameters such as biomechanical, demographic and genetic data. In order to achieve this, we will train cutting edge machine learning techniques on the Moorfields Corneal Bioresource (MCB), a large database that combines detailed longitudinal imaging data (since 2009) linked to clinical notes and recently acquired genetic data from over 3000 patients who have attended refractive and KC clinics.
Progression
The ability to detect and model disease progression is extremely important when deciding the appropriate course of treatment. Very little has been published concerning the application of machine learning methods to identify keratoconus progression. We hope to advance research in this field by applying techniques such as recurrent neural networks to detect and model disease progression over time. We also hope to build visualization tools to aid clinicians understand the evolution of corneal morphology over time.
Treatment
Once a progressive keratoconus diagnosis has been established, the next key part of the clinical pathway is to decide the course of optimal treatment. Our team will use the extensive raw patient data from Moorfields Eye Hospital to create rich training datasets linking images, corneal parameters, patient attributes, treatment data, treatment outcomes and genetic data. Machine learning models will be trained on the resulting dataset to predict post-treatment outcomes along with identifying clinical, imaging or genetic risk factors. This could take the form of supervised learning if only categorical or imaging data is analysed or unsupervised learning in the case of genetic data.